Analysis of batching strategies for multi-item production with yield uncertainty
Author(s)
Siow, Christopher (Christopher Shun Yi)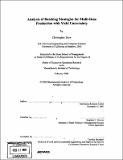
DownloadFull printable version (5.911Mb)
Other Contributors
Massachusetts Institute of Technology. Operations Research Center.
Advisor
Stephen C. Graves.
Terms of use
Metadata
Show full item recordAbstract
In this thesis, we investigate the batch sizing problem for a custom-job production facility. More specifically, given a production system that has been assigned several different types of custom jobs, we try to derive batching policies to minimize the expected total time that a job spends in the system. Custom-job production brings a host of challenges that makes batch sizing very difficult - production can only begin when the order arrives, the yield uncertainty probabilities are fairly large, and the production quantities are typically small. Furthermore, deriving an optimal batch sizing policy is difficult due to the heterogeneity of the job types; each job type has a different demand, batch setup time, unit production rate, unit defective probability, and job arrival rate. In addition, further complexity stems from the fact that the batch sizing decisions for each job type are coupled, and cannot be made independently. Given the difficulties in selecting the batch sizes, we propose an alternative batching method that minimizes the system utilization instead of the expected total job time. The main advantage of this approach is that is allows us to choose the batch size of each job type individually. First, we model the system as an M/G/l queue, and obtain a closed-form expression for the expected total job time when the demand is restricted to be a single unit. Following which, we show empirically that the minimum utilization heuristic attains near-optimal performance under the unit demand restriction. We then build on this analysis, and extend the heuristic to the general case in which the demand of each job is allowed to be more than a single unit. Finally, we use simulations to compare our heuristic against other alternative batching policies, and the results indicate that our heuristic is indeed an effective strategy.
Description
Thesis (S.M.)--Massachusetts Institute of Technology, Sloan School of Management, Operations Research Center, 2008. Includes bibliographical references (p. 179-180).
Date issued
2008Department
Massachusetts Institute of Technology. Operations Research Center; Sloan School of ManagementPublisher
Massachusetts Institute of Technology
Keywords
Operations Research Center.