Nonparametric Bayesian behavior modeling
Author(s)
Joseph, Joshua Mason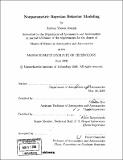
DownloadFull printable version (18.36Mb)
Other Contributors
Massachusetts Institute of Technology. Dept. of Aeronautics and Astronautics.
Advisor
Nicholas Roy and Adam Rzepniewski.
Terms of use
Metadata
Show full item recordAbstract
As autonomous robots are increasingly used in complex, dynamic environments, it is crucial that the dynamic elements are modeled accurately. However, it is often difficult to generate good models due to either a lack of domain understanding or the domain being intractably large. In many domains, even defining the size of the model can be a challenge. While methods exist to cluster data of dynamic agents into common motion patterns, or "behaviors," assumptions of the number of expected behaviors must be made. This assumption can cause clustering processes to under-fit or over-fit the training data. In a poorly understood domain, knowing the number of expected behaviors a priori is unrealistic and in an extremely large domain, correctly fitting the training data is difficult. To overcome these obstacles, this thesis takes a Bayesian approach and applies a Dirichlet process (DP) prior over behaviors, which uses experience to reduce the likelihood of over-fitting or under-fitting the model complexity. Additionally, the DP maintains a probability mass associated with a novel behavior and can address countably infinite behaviors. This learning technique is applied to modeling agents driving in an urban setting. The learned DP-based driver behavior model is first demonstrated on a simulated city. Building on successful simulation results, the methodology is applied to GPS data of taxis driving around Boston. Accurate prediction of future vehicle behavior from the model is shown in both domains.
Description
Thesis (S.M.)--Massachusetts Institute of Technology, Dept. of Aeronautics and Astronautics, 2008. Includes bibliographical references (p. 91-94).
Date issued
2008Department
Massachusetts Institute of Technology. Department of Aeronautics and AstronauticsPublisher
Massachusetts Institute of Technology
Keywords
Aeronautics and Astronautics.