Techniques to account for and reduce model inadequacy in ensemble-based filters
Author(s)
Khade, Vikram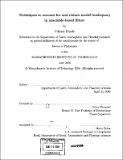
DownloadFull printable version (35.48Mb)
Other Contributors
Massachusetts Institute of Technology. Dept. of Earth, Atmospheric, and Planetary Sciences.
Advisor
Kerry Emmanuel.
Terms of use
Metadata
Show full item recordAbstract
A technique for the accounting for parametric model error in the Ensemble Kalman Filter (EnKF) is investigated within the framework of Additive Error Approximation (AEA). The AEA needs an estimate of the model error covariance structure. The state-dependent model error structure is the sensitivity of the local attractor to the parameter. The Multimodel Method (MMM) and Parametric Vector Method (PVM) to estimate this state-dependent sensitivity are introduced and investigated in the low-dimensional Ikeda and L63 systems. The MMM involves assimilating data independently into multiple models. PVM aims at obtaining the estimate given by MMM using a single model. At the heart of the PVM is the concept of adjoint sensitivity which is obtained using parametric singular vectors. It is found that PVM is able to estimate the correct state-dependent model error structure if the parametric vectors are constructed over an optimization time (T0p) which is equal to the state-dependent optimal time (Tm). The optimal time is the time taken by a state to go from an off- attractor location to an on-attractor location. If Top < rm then the parametric vector gives the transient sensitivity which is the incorrect model error structure. On the other hand, if rp > Tom the sensitivity obtained is non-local and tends to point in the direction of largest state error growth. The average (over the phase space) Tom is calculated for the Ikeda and L63 systems. MMM and PVM give lower average analysis and forecast errors than state-independent estimates of model error structure. Parameter estimation is a typical example of reduction of model error. The state-dependent parameter estimation (parameter tuning) in the Ikeda system is successful in partially compensating for structural model error thus resulting in lower analysis and forecast errors. However, parameter tuning is not able to completely eliminate structural model error. Nonetheless, parameter tuning can be used to identify processes in the model that have large model error. The parameters in the Emanuel convection scheme are tuned in the NOGAPS model. This parameter tuning is able to partially compensate for structural model error in the vertical flux parametrization.
Description
Thesis (Ph. D.)--Massachusetts Institute of Technology, Dept. of Earth, Atmospheric, and Planetary Sciences, 2008. Includes bibliographical references (p. 133-136).
Date issued
2008Department
Massachusetts Institute of Technology. Department of Earth, Atmospheric, and Planetary SciencesPublisher
Massachusetts Institute of Technology
Keywords
Earth, Atmospheric, and Planetary Sciences.