Learning for informative path planning
Author(s)
Park, Sooho, S.M. Massachusetts Institute of Technology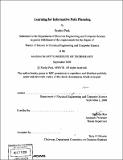
DownloadFull printable version (16.13Mb)
Other Contributors
Massachusetts Institute of Technology. Dept. of Electrical Engineering and Computer Science.
Advisor
Nicholas Roy.
Terms of use
Metadata
Show full item recordAbstract
Through the combined use of regression techniques, we will learn models of the uncertainty propagation efficiently and accurately to replace computationally intensive Monte- Carlo simulations in informative path planning. This will enable us to decrease the uncertainty of the weather estimates more than current methods by enabling the evaluation of many more candidate paths given the same amount of resources. The learning method and the path planning method will be validated by the numerical experiments using the Lorenz-2003 model [32], an idealized weather model.
Description
Thesis (S.M.)--Massachusetts Institute of Technology, Dept. of Electrical Engineering and Computer Science, 2008. Includes bibliographical references (p. 104-108).
Date issued
2008Department
Massachusetts Institute of Technology. Department of Electrical Engineering and Computer SciencePublisher
Massachusetts Institute of Technology
Keywords
Electrical Engineering and Computer Science.