Ensemble regression : using ensemble model output for atmospheric dynamics and prediction
Author(s)
Gombos, Daniel (Daniel Lawrence)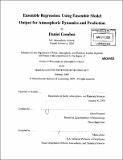
DownloadFull printable version (25.61Mb)
Alternative title
Using ensemble model output for atmospheric dynamics and prediction
Other Contributors
Massachusetts Institute of Technology. Dept. of Earth, Atmospheric, and Planetary Sciences.
Advisor
Kerry Emanuel.
Terms of use
Metadata
Show full item recordAbstract
Ensemble regression (ER) is a linear inversion technique that uses ensemble statistics from atmospheric model output to make dynamical inferences and forecasts. ER defines a multivariate regression operator using ensemble forecasts and analyses to determine the most probable predict and perturbation associated with the prescribed predictor perturbation resolved by linear combinations of the predictor ensemble anomalies. Because it employs flow-dependent ensemble data, as opposed to the stationary time series data typically used to make statistical forecasts, ER is capable of modeling synoptic scale processes with rapidly evolving covariances. This characteristic is applied in several ways. Firstly, it is shown that the classical dynamical piecewise potential vorticity (PV) inversion of the PV perturbation effectively resolved by the ER operator yields nearly identical geopotential heights to those deduced from an ER performed in the subspace of the leading PV singular vectors. Secondly, using the example of the lagged sensitivity of tropical cyclone tracks to preexisting midtropospheric heights, ER is used to infer dynamical relationships from statistical sensitivities, to identify, in real-time, the dynamical processes that are particularly relevant to specific forecast decisions, and to make preemptive forecasts. Thirdly, it is shown that singular vectors deduced from the ER operator approximate those from the analysis error covariance normed tangent linear model operator, suggesting a simple alternative method for computing singular vectors. Given that ER results are a function of forecast ensemble reliability, theory and applications of a multivariate ensemble reliability verification technique called the minimum spanning tree rank histogram are presented. (cont.) Experiments using Euclidean, variance, and Mahalanobis norms for defining minimum spanning tree distances imply that, unless the number of ensemble members is less than or equal to the number of dimensions being verified, the Mahalanobis norm transforms a spanning tree into a space where model imperfections are most readily identified.
Description
Thesis (Ph. D.)--Massachusetts Institute of Technology, Dept. of Earth, Atmospheric, and Planetary Sciences, 2009. Includes bibliographical references (p. 183-190).
Date issued
2009Department
Massachusetts Institute of Technology. Department of Earth, Atmospheric, and Planetary SciencesPublisher
Massachusetts Institute of Technology
Keywords
Earth, Atmospheric, and Planetary Sciences.