Model Reduction for Dynamic Sensor Steering: A Bayesian Approach to Inverse Problems
Author(s)
Wogrin, Sonja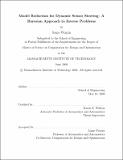
DownloadACDL_TR-08-3.pdf (1.815Mb)
Metadata
Show full item recordAbstract
In many settings, distributed sensors provide dynamic measurements over a specified time horizon that can be used to reconstruct information such as parameters, states or initial conditions. This estimation task can be posed formally as an inverse problem: given a model and a set of measurements, estimate the parameters of interest. We consider the specific problem of computing in real-time the prediction of a contamination event, based on measurements obtained by mobile sensors. The spread of the contamination is modeled by the convection diffusion equation. A Bayesian approach to the inverse problem yields an estimate of the probability density function of the initial contaminant concentration, which can then be propagated through the forward model to determine the predicted contaminant field at some future time and its associated uncertainty distribution. Sensor steering is effected by formulating and solving an optimization problem that seeks the sensor locations that minimize the uncertainty in this prediction. An important aspect of this Dynamic Sensor Steering Algorithm is the ability to execute in real-time. We achieve this through reduced-order modeling, which (for our two-dimensional examples) yields models that can be solved two orders of magnitude faster than the original system, but only incur average relative errors of magnitude O(10−3). The methodology is demonstrated on the contaminant transport problem, but is applicable to a broad class of problems where we wish to observe certain phenomena whose location or features are not known a priori.
Date issued
2008-06Publisher
Aerospace Computational Design Laboratory, Dept. of Aeronautics & Astronautics, Massachusetts Institute of Technology
Series/Report no.
ACDL Technical Reports;ACDL TR-08-3