A regularization framework for active learning from imbalanced data
Author(s)
Paskov, Hristo Spassimirov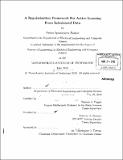
DownloadFull printable version (6.283Mb)
Alternative title
Multiclass extensions of Regularized Least Squares
Other Contributors
Massachusetts Institute of Technology. Dept. of Electrical Engineering and Computer Science.
Advisor
Tomaso A. Poggio and Lorenzo A. Rosasco.
Terms of use
Metadata
Show full item recordAbstract
We consider the problem of building a viable multiclass classification system that minimizes training data, is robust to noisy, imbalanced samples, and outputs confidence scores along with its predications. These goals address critical steps along the entire classification pipeline that pertain to collecting data, training, and classifying. To this end, we investigate the merits of a classification framework that uses a robust algorithm known as Regularized Least Squares (RLS) as its basic classifier. We extend RLS to account for data imbalances, perform efficient active learning, and output confidence scores. Each of these extensions is a new result that combines with our other findings to give an altogether novel and effective classification system. Our first set of results investigates various ways to handle multiclass data imbalances and ultimately leads to a derivation of a weighted version of RLS with and without an offset term. Weighting RLS provides an effective countermeasure to imbalanced data and facilitates the automatic selection of a regularization parameter through exact and efficient calculation of the Leave One Out error. Next, we present two methods that estimate multiclass confidence from an asymptotic analysis of RLS and another method that stems from a Bayesian interpretation of the classifier. We show that while the third method incorporates more information in its estimate, the asymptotic methods are more accurate and resilient to imperfect kernel and regularization parameter choices. Finally, we present an active learning extension of RLS (ARLS) that uses our weighting methods to overcome imbalanced data. ARLS is particularly adept to this task because of its intelligent selection scheme.
Description
Thesis (M. Eng.)--Massachusetts Institute of Technology, Dept. of Electrical Engineering and Computer Science, 2010. Cataloged from PDF version of thesis. Includes bibliographical references (p. 81-83).
Date issued
2010Department
Massachusetts Institute of Technology. Department of Electrical Engineering and Computer SciencePublisher
Massachusetts Institute of Technology
Keywords
Electrical Engineering and Computer Science.