Designing an error resolution checklist for a shared manned-unmanned environment
Author(s)
Tappan, Jacqueline M. (Jacqueline Marie)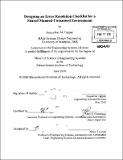
DownloadFull printable version (16.62Mb)
Other Contributors
Massachusetts Institute of Technology. Engineering Systems Division.
Advisor
Mary L. Cummings.
Terms of use
Metadata
Show full item recordAbstract
The role of unmanned vehicles in military and commercial environments continues to expand, resulting in Shared Manned-Unmanned (SMU) domains. While the introduction of unmanned vehicles can have many benefits, humans operating within these environments must shift to high-level supervisory roles, which will require them to resolve system errors. Error resolution in current Human Supervisory Control (HSC) domains is performed using a checklist; the error is quickly identified, and then resolved using the steps outlined by the checklist. Background research into error resolution identified three attributes that impact the effectiveness of an error resolution checklist: domain predictability, sensor reliability, and time availability. These attributes were combined into a Checklist Attribute Model (CAM), demonstrating that HSC domains with high levels of complexity (e.g. SMU domains) are ill-suited to error resolution using traditional checklists. In particular, it was found that more support was required during such error identification, as data is uncertain and unreliable. A new error resolution checklist, termed the GUIDER (Graphical User Interface for Directed Error Recovery) Probabilistic Checklist, was developed to aid the human during the error identification process in SMU domains. Evaluation was performed through a human performance experiment requiring participants to resolve errors in a simulated SMU domain using the GUIDER Probabilistic Checklist and a traditional checklist tool. Thirty-six participants were recruited, and each was assigned to a single checklist tool condition. Participants completed three simulated error scenarios. The three scenarios had varying sensor reliability levels (low, medium, high) to gauge the impact of uncertainty on the usefulness of each checklist tool. The human performance experiment showed that the addition of error likelihood data using an intuitive visualization through the GUIDER Probabilistic Checklist improved error resolution in uncertain settings. In settings with high certainty, there was no difference found between the performances of the two checklists. While positive, further testing is required in more realistic settings to validate both the effectiveness of the GUIDER Probabilistic Checklist tool and the Checklist Attribute Model.
Description
Thesis (S.M.)--Massachusetts Institute of Technology, Engineering Systems Division, 2010. Cataloged from PDF version of thesis. Includes bibliographical references (p. 151-153).
Date issued
2010Department
Massachusetts Institute of Technology. Engineering Systems DivisionPublisher
Massachusetts Institute of Technology
Keywords
Engineering Systems Division.