Adaptive models for the recognition of human gesture
Author(s)
Wilson, Andrew David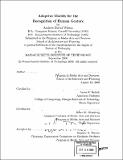
DownloadFull printable version (13.31Mb)
Other Contributors
Massachusetts Institute of Technology. Dept. of Architecture. Program In Media Arts and Sciences.
Advisor
Aaron F. Bobick and Bruce M. Blumberg.
Terms of use
Metadata
Show full item recordAbstract
Tomorrow's ubiquitous computing environments will go beyond the keyboard, mouse and monitor paradigm of interaction and will require the automatic interpretation of human motion using a variety of sensors including video cameras. I present several techniques for human motion recognition that are inspired by observations on human gesture, the class of communicative human movement. Typically, gesture recognition systems are unable to handle systematic variation in the input signal, and so are too brittle to be applied successfully in many real-world situations. To address this problem, I present modeling and recognition techniques to adapt gesture models to the situation at hand. A number of systems and frameworks that use adaptive gesture models are presented. First, the parametric hidden Markov model (PHMM) addresses the representation and recognition of gesture families, to extract how a gesture is executed. Second, strong temporal models drawn from natural gesture theory are exploited to segment two kinds of natural gestures from video sequences. Third, a realtime computer vision system learns gesture models online from time-varying context. Fourth, a realtime computer vision system employs hybrid Bayesian networks to unify and extend the previous approaches, as well as point the way for future work.
Description
Thesis (Ph.D.)--Massachusetts Institute of Technology, School of Architecture and Planning, Program in Media Arts and Sciences, 2000. Includes bibliographical references (leaves 135-140).
Date issued
2000Department
Program in Media Arts and Sciences (Massachusetts Institute of Technology)Publisher
Massachusetts Institute of Technology
Keywords
Architecture. Program In Media Arts and Sciences.