Large-deviation analysis and applications Of learning tree-structured graphical models
Author(s)
Tan, Vincent Yan Fu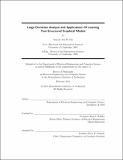
DownloadFull printable version (1.862Mb)
Other Contributors
Massachusetts Institute of Technology. Dept. of Electrical Engineering and Computer Science.
Advisor
Alan S. Willsky.
Terms of use
Metadata
Show full item recordAbstract
The design and analysis of complexity-reduced representations for multivariate data is important in many scientific and engineering domains. This thesis explores such representations from two different perspectives: deriving and analyzing performance measures for learning tree-structured graphical models and salient feature subset selection for discrimination. Graphical models have proven to be a flexible class of probabilistic models for approximating high-dimensional data. Learning the structure of such models from data is an important generic task. It is known that if the data are drawn from tree-structured distributions, then the algorithm of Chow and Liu (1968) provides an efficient algorithm for finding the tree that maximizes the likelihood of the data. We leverage this algorithm and the theory of large deviations to derive the error exponent of structure learning for discrete and Gaussian graphical models. We determine the extremal tree structures for learning, that is, the structures that lead to the highest and lowest exponents. We prove that the star minimizes the exponent and the chain maximizes the exponent, which means that among all unlabeled trees, the star and the chain are the worst and best for learning respectively. The analysis is also extended to learning foreststructured graphical models by augmenting the Chow-Liu algorithm with a thresholding procedure. We prove scaling laws on the number of samples and the number variables for structure learning to remain consistent in high-dimensions. The next part of the thesis is concerned with discrimination. We design computationally efficient tree-based algorithms to learn pairs of distributions that are specifically adapted to the task of discrimination and show that they perform well on various datasets vis-`a-vis existing tree-based algorithms. We define the notion of a salient set for discrimination using information-theoretic quantities and derive scaling laws on the number of samples so that the salient set can be recovered asymptotically.
Description
Thesis (Ph. D.)--Massachusetts Institute of Technology, Dept. of Electrical Engineering and Computer Science, 2011. This electronic version was submitted by the student author. The certified thesis is available in the Institute Archives and Special Collections. Cataloged from student submitted PDF version of thesis. Includes bibliographical references (p. 213-228).
Date issued
2011Department
Massachusetts Institute of Technology. Department of Electrical Engineering and Computer SciencePublisher
Massachusetts Institute of Technology
Keywords
Electrical Engineering and Computer Science.