Learning visual object categories from few training examples
Author(s)
Kuo, Michael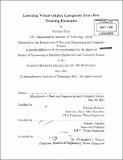
DownloadFull printable version (13.83Mb)
Other Contributors
Massachusetts Institute of Technology. Dept. of Electrical Engineering and Computer Science.
Advisor
Predrag Neskovic and Antonio Torralba.
Terms of use
Metadata
Show full item recordAbstract
During visual perception of complex objects, humans fixate on salient regions of a particular object, moving their gaze from one region to another in order to gain information about that object. The Bayesian Integrate and Shift (BIAS) model is a recently proposed model for learning visual object categories that is modeled after the process of human visual perception, integrating information from within and across fixations. Previous works have described preliminary evaluations of the BIAS model and demonstrated that it can learn new object categories from only a few examples. In this thesis, we introduce and evaluate improvements to the learning algorithm, demonstrate that the model benefits from using information from fixating on multiple regions of a particular object, evaluate the limitations of the model when learning different object categories, and assess the performance of the learning algorithm when objects are partially occluded.
Description
Thesis (M. Eng.)--Massachusetts Institute of Technology, Dept. of Electrical Engineering and Computer Science, 2011. Cataloged from PDF version of thesis. Includes bibliographical references (p. 73-74).
Date issued
2011Department
Massachusetts Institute of Technology. Department of Electrical Engineering and Computer SciencePublisher
Massachusetts Institute of Technology
Keywords
Electrical Engineering and Computer Science.