Labeling and modeling large databases of videos
Author(s)
Yuen, Jenny, Ph. D. Massachusetts Institute of Technology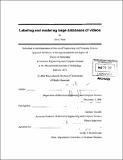
DownloadFull printable version (11.13Mb)
Other Contributors
Massachusetts Institute of Technology. Dept. of Electrical Engineering and Computer Science.
Advisor
Antonio Torralba.
Terms of use
Metadata
Show full item recordAbstract
As humans, we can say many things about the scenes surrounding us. For instance, we can tell what type of scene and location an image depicts, describe what objects live in it, their material properties, or their spatial arrangement. These comprise descriptions of a scene and are majorly studied areas in computer vision. This thesis, however, hypotheses that observers have an inherent prior knowledge that can be applied to the scene at hand. This prior knowledge can be translated into the cognisance of which objects move, or in the trajectories and velocities to expect. Conversely, when faced with unusual events such as car accidents, humans are very well tuned to identify them regardless of having observed the scene a priori. This is, in part, due to prior observations that we have for scenes with similar configurations to the current one. This thesis emulates the prior knowledge base of humans by creating a large and heterogeneous database and annotation tool for videos depicting real world scenes. The first application of this thesis is in the area of unusual event detection. Given a short clip, the task is to identify the moving portions of the scene that depict abnormal events. We adopt a data-driven framework powered by scene matching techniques to retrieve the videos nearest to the query clip and integrate the motion information in the nearest videos. The result is a final clip with localized annotations for unusual activity. The second application lies in the area of event prediction. Given a static image, we adapt our framework to compile a prediction of motions to expect in the image. This result is crafted by integrating the knowledge of videos depicting scenes similar to the query image. With the help of scene matching, only scenes relevant to the queries are considered, resulting in reliable predictions. Our dataset, experimentation, and proposed model introduce and explore a new facet of scene understanding in images and videos.
Description
Thesis (Ph. D.)--Massachusetts Institute of Technology, Dept. of Electrical Engineering and Computer Science, 2012. Cataloged from PDF version of thesis. Includes bibliographical references (p. 91-98).
Date issued
2012Department
Massachusetts Institute of Technology. Department of Electrical Engineering and Computer SciencePublisher
Massachusetts Institute of Technology
Keywords
Electrical Engineering and Computer Science.