A chronological probabilistic production cost model to evaluate the reliability contribution of limited energy plants
Author(s)
Leung, Tommy (Tommy Chun Ting)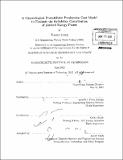
DownloadFull printable version (6.842Mb)
Other Contributors
Massachusetts Institute of Technology. Technology and Policy Program.
Advisor
Ignacio J. Prez Arriaga and Carlos Batlle.
Terms of use
Metadata
Show full item recordAbstract
The growth of renewables in power systems has reinvigorated research and regulatory interest in reliability analysis algorithms such as the Baleriaux/Booth convolution-based probabilistic production cost (PPC) model. However, while these traditional PPC algorithms can reasonably represent thermal plant availabilities, they do not accurately represent limited energy plants because of their generic treatment of time. In particular, in systems with limited energy plants, convolution-based PPC models tend to underestimate the loss-of-load probability and expected nonserved energy. This thesis illustrates the chronological challenges of the traditional convolution-based PPC, proposes a modification that improves the representation of chronological elements, explores the reliability contribution of LEPs using the new algorithm, and demonstrates two regulatory applications by calculating a capacity payment for an LEP and the expected-load-carrying-capability metric for any generator. To the best knowledge of the author, the introduction of multiple hydro plants with different capacity constraints and the calculations for marginal probabilities, prices, and revenues to a chronological PPC model are novel.
Description
Thesis (S.M. in Technology and Policy)-- Massachusetts Institute of Technology, Engineering Systems Division, Technology and Policy Program, 2012. Cataloged from PDF version of thesis. Includes bibliographical references (p. 73-74).
Date issued
2012Department
Massachusetts Institute of Technology. Engineering Systems Division; Technology and Policy ProgramPublisher
Massachusetts Institute of Technology
Keywords
Engineering Systems Division., Technology and Policy Program.