Aggregation and influence in teams of imperfect decision makers
Author(s)
Rhim, Joong Bum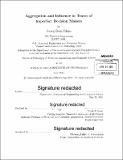
DownloadFull printable version (7.611Mb)
Other Contributors
Massachusetts Institute of Technology. Department of Electrical Engineering and Computer Science.
Advisor
Vivek K Goyal.
Terms of use
Metadata
Show full item recordAbstract
Bayesian hypothesis testing inevitably requires prior probabilities of hypotheses. Motivated by human decision makers, this thesis studies how binary decision making is performed when the decision-making agents use imperfect prior probabilities. Three detection models with multiple agents are investigated: distributed detection with symmetric fusion, sequential detection with social learning, and distributed detection with symmetric fusion and social learning. In the distributed detection with symmetric fusion, we consider the agents to be a team aiming to minimize the Bayes risk of the team's decision. In this model, incorrect beliefs reduce the chance of the agents from being right so always lead to an increase in the Bayes risk of the decision-making team. In contrast, the role of beliefs is more complicated in the sequential detection model with social learning, where agents observe public signals, which are decisions made by other agents. Since each agent affects the minimum possible Bayes risk for subsequent agents, she may have a mixed objective including her own Bayes risk and the Bayes risks of subsequent agents. For an earlier-acting agent, it is shown that being informative to later-acting agents is different from being right. When private signals are described by Gaussian likelihoods, informative earlier-acting agents should be open-minded toward the unlikely hypothesis. Social learning helps imperfect agents who have favorable incorrect beliefs outperform perfect agents who have correct beliefs. Compared to in the sequential detection model, social learning is less influential in the distributed detection model with symmetric fusion. This is because social learning induces the evolution of the fusion rule in the distributed detection model, which countervails the other effect of social learning-belief update. In particular, social learning is futile when the agents observe conditionally independent and identically distributed private signals or when the agents require unanimity to make a decision. Since social learning is ineffective, imperfect agents cannot outperform perfect agents, unlike in the sequential detection model. Experiments about human behavior were performed in team decision-making situations when people should optimally ignore public signals. The experiments suggest that when people vote with equal qualities of information, the ballots should be secret.
Description
Thesis: Ph. D., Massachusetts Institute of Technology, Department of Electrical Engineering and Computer Science, 2014. Cataloged from PDF version of thesis. Includes bibliographical references (pages 137-141).
Date issued
2014Department
Massachusetts Institute of Technology. Department of Electrical Engineering and Computer SciencePublisher
Massachusetts Institute of Technology
Keywords
Electrical Engineering and Computer Science.