Predictive storm damage modeling and optimizing crew response to improve storm response operations
Author(s)
Whipple, Sean David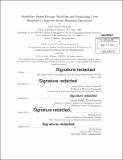
DownloadFull printable version (4.786Mb)
Alternative title
Predictive storm damage modeling and repair crew optimization
Other Contributors
Leaders for Global Operations Program.
Advisor
James Kirtley and Georgia Perakis.
Terms of use
Metadata
Show full item recordAbstract
Utility infrastructures are constantly damaged by naturally occurring weather. Such damage results in customer service interruption and repairs are necessary to return the system to normal operation. In most cases these events are few and far between but major storm events (i.e. Hurricane Sandy) cause damage on a significantly higher scale. Large numbers of customers have service interrupted and repair costs are in the millions of dollars. The ability to predict damage before the event and optimize response can significantly cut costs. The first task was to develop a model to predict outages on the network. Using weather data from the past six storms as well as outage data from the events, asset information (framing, pole age, etc.), and environmental information were used to understand the interactions that lead to outages (forested areas are more likely to have outages than underground assets for example). Utilizing data mining and machine learning techniques we developed a model that gathers the data and applies a classification tree model to predict outages caused by weather. Next we developed an optimization model to allocate repair crews across Atlantic Electric staging locations in response to the predicted damage to ensure the earliest possible restoration time. Regulators impose constraints such as cost and return to service time on utility firms and these constraints will largely drive the distribution of repair crews. While the model starts with predicted results, the use of robust optimization will allow Atlantic Electric to optimize their response despite the uncertainty of why outages have occurred, which will lead to more effective response planning and execution across a variety of weather-related outages. Using these models Atlantic Electric will have data driven capability to not only predict how much damage an incoming storm will produce, but also aid in planning how to allocate their repair crews. These tools will ensure Atlantic Electric can properly plan for storm events and as more storms occur the tools will increase their efficacy.
Description
Thesis: S.M., Massachusetts Institute of Technology, Engineering Systems Division, 2014. In conjunction with the Leaders for Global Operations Program at MIT. Thesis: M.B.A., Massachusetts Institute of Technology, Sloan School of Management, 2014. In conjunction with the Leaders for Global Operations Program at MIT. Cataloged from PDF version of thesis. Includes bibliographical references (pages 61-63).
Date issued
2014Department
Leaders for Global Operations Program at MIT; Massachusetts Institute of Technology. Engineering Systems Division; Sloan School of ManagementPublisher
Massachusetts Institute of Technology
Keywords
Engineering Systems Division., Sloan School of Management., Leaders for Global Operations Program.