A Bayesian framework for fracture characterization from surface seismic data
Author(s)
Zamanian, S. Ahmad; Fehler, Michael; Burns, Daniel R.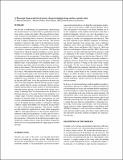
DownloadZamanian-Fehler-Burns_Bayesian framework for fracture.pdf (331.8Kb)
Other Contributors
Massachusetts Institute of Technology. Earth Resources Laboratory
Metadata
Show full item recordAbstract
We describe a methodology for quantitatively characterizing the fractured nature of a hydrocarbon or geothermal reservoir from surface seismic data under a Bayesian inference framework. Fractures provide pathways for fluid flow in a reservoir, and hence, knowledge about a reservoir’s fractured nature can be used to enhance production of the reservoir. The fracture properties of interest in this study (to be inferred) are fracture orientation and excess compliance, where each of these properties are assumed to vary spatially over a 2D lateral grid which is assumed to represent the top of a reservoir. The Bayesian framework in which the inference problem is cast has the key benefits of (1) utilization of a prior model that allows geological information to be incorporated, (2) providing a straightforward means of incorporating all measurements (across the 2D spatial grid) into the estimates at each grid point, (3) allowing different types of measurements to be combined under a single inference procedure, and (4) providing a measure of uncertainty in the estimates. The observed data are taken from a 2D array of surface seismic receivers responding to an array of surface sources. Well understood features from the seismic traces are extracted and treated as the observed data, namely the P-wave reflection amplitude variation with acquisition azimuth (amplitude versus azimuth, or AvAz, data) and fracture transfer function (FTF) data. AvAz data are known to be more sensitive to fracture properties when the fracture spacing is significantly smaller than the seismic wavelength, whereas fracture transfer function data are more sensitive to fracture properties when the fracture spacing is on the order of the seismic wavelength. Combining these two measurements has the benefit of allowing inferences to be made about fracture properties over a larger range of fracture spacing than otherwise attainable. Geophysical forward models for the measurements are used to arrive at likelihood models for the data. The prior distribution for the hidden fracture variables is obtained by defining a Markov random field (MRF) over the lateral 2D grid where we wish to obtain fracture properties. The fracture variables are then inferred by application of loopy belief propagation (LBP) to yield approximations for the posterior marginal distributions of the fracture properties, as well as the maximum a posteriori (MAP) and Bayes least squares (BLS) estimates of these properties. Verification of the inference procedure is performed on a synthetic dataset, where the estimates obtained are shown to be at or near ground truth for a large range of fracture spacings.
Date issued
2012Publisher
Massachusetts Institute of Technology. Earth Resources Laboratory
Series/Report no.
Earth Resources Laboratory Industry Consortia Annual Report;2012-05
Keywords
Fractures, Geothermal, Modeling, Inversion