Improving inbound visibility through shipment arrival modeling
Author(s)
Chun, Michael (Michael M.)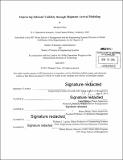
DownloadFull printable version (5.063Mb)
Other Contributors
Leaders for Global Operations Program.
Advisor
Edgar Blanco and Karen Zheng.
Terms of use
Metadata
Show full item recordAbstract
Amazon's desire to provide the "Earth's largest selection" comes at a tremendous cost. In addition to managing the orders for thousands of vendors/suppliers and millions of Stock Keeping Units (SKUs), Amazon must keep track of all the inbound shipments in order to manage its inventory efficiently. Although estimated delivery dates are routinely received for each of these inbound shipments, only about half of the purchase orders actually arrives by these dates. Since knowing exactly how much to order is based in part by what has already been ordered in the past and when those shipments will arrive, this inaccuracy makes determining optimal purchase order quantities difficult for future orders. So in order to optimize the inbound process, Amazon must either improve the accuracy of these estimates or account for the inherent variation. This thesis establishes a model that exposes the underlying variation for each inbound arrival signal based on historical error rates. Our approach is to map all inbound signal sources and then create a classification-tr-e model that minimizes the joint variance of the prediction errors. Simulations indicate that such a model can be used to generate new estimated arrival dates that reflect the likelihood of arrival. In addition, this thesis also takes a step further to outline some potential vendor policy changes for eliminating the root causes of procurement lead time variance.
Description
Thesis: M.B.A., Massachusetts Institute of Technology, Sloan School of Management, 2014. In conjunction with the Leaders for Global Operations Program at MIT. Thesis: S.M., Massachusetts Institute of Technology, Engineering Systems Division, 2014. In conjunction with the Leaders for Global Operations Program at MIT. Cataloged from PDF version of thesis. Includes bibliographical references (pages 48-49).
Date issued
2014Department
Leaders for Global Operations Program at MIT; Massachusetts Institute of Technology. Engineering Systems Division; Sloan School of ManagementPublisher
Massachusetts Institute of Technology
Keywords
Sloan School of Management., Engineering Systems Division., Leaders for Global Operations Program.