Joint multilingual learning for coreference resolution
Author(s)
Bodnari, Andreea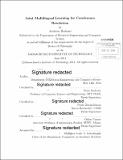
DownloadFull printable version (16.99Mb)
Other Contributors
Massachusetts Institute of Technology. Department of Electrical Engineering and Computer Science.
Advisor
Peter Szolovits, Pierre Zweigenbaum, and Özlem Uzuner.
Terms of use
Metadata
Show full item recordAbstract
Natural language is a pervasive human skill not yet fully achievable by automated computing systems. The main challenge is understanding how to computationally model both the depth and the breadth of natural languages. In this thesis, I present two probabilistic models that systematically model both the depth and the breadth of natural languages for two different linguistic tasks: syntactic parsing and joint learning of named entity recognition and coreference resolution. The syntactic parsing model outperforms current state-of-the-art models by discovering linguistic information shared across languages at the granular level of a sentence. The coreference resolution system is one of the first attempts at joint multilingual modeling of named entity recognition and coreference resolution with limited linguistic resources. It performs second best on three out of four languages when compared to state-of-the-art systems built with rich linguistic resources. I show that we can simultaneously model both the depth and the breadth of natural languages using the underlying linguistic structure shared across languages.
Description
Thesis: Ph. D., Massachusetts Institute of Technology, Department of Electrical Engineering and Computer Science, 2014. 98 Cataloged from PDF version of thesis. Includes bibliographical references (pages 112-120).
Date issued
2014Department
Massachusetts Institute of Technology. Department of Electrical Engineering and Computer SciencePublisher
Massachusetts Institute of Technology
Keywords
Electrical Engineering and Computer Science.