Spatiotemporal learning and geo-visualization methods for constructing activity-travel patterns from transit card transaction data
Author(s)
Zhu, Yi, Ph. D. Massachusetts Institute of Technology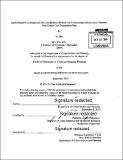
DownloadFull printable version (29.43Mb)
Other Contributors
Massachusetts Institute of Technology. Department of Urban Studies and Planning.
Advisor
Joseph Ferreira, Jr.
Terms of use
Metadata
Show full item recordAbstract
The study of human activity-travel patterns for transportation demand forecast has evolved a long way in theories, methodologies and applications. However, the scarcity of data has become a major barrier for the advancement of research in the field. At the same time, the proliferation of urban sensing and location-based devices generate voluminous streams of spatio-temporal registered information. These urban sensing data contain massive information on urban dynamics and individuals' mobility. For example, the transit smart card transaction data reveal the places that transit passengers visit at different times of day. As tempting as it appears to be, the incorporation of these urban sensing data into activity-travel study remains a big challenge, which demands new analytics, theories and frameworks to bridge the gap between the information observed directly from the imperfect urban sensing data and the knowledge about how people use the city. In this study, we propose a framework of analysis that focuses on the recurring processing and learning of voluminous transit smart card data flows in juxtaposition with additional auxiliary spatio-temporal data, which are used to improve our understanding of the context of the data. The framework consists of an ontology-based data integration process, a built environment measurement module, an activity-learning module and visualization examples that facilitate the exploration and investigation of activity-travel patterns. The ontology-based data integration approach helps to integrate and interpret spatio-temporal data from multiple sources in a systematic way. These spatio-temporally detailed data are used to formulate quantitative variables for the characterization of the context under which the travelers made their transit trips. In particular, a set of spatial metrics are computed to measure different dimensionalities of the urban built environment of trip destinations. In order to understand why people make trips to destinations, researchers and planners need to know the possible activities associated with observed transit trips. Therefore, an activity learning module is developed to infer the unknown activity types from millions of trips recorded in transit smart card transactions by learning the context dependent behaviors of travelers from a traditional household travel survey. The learned activities not only help the interpretation of the behavioral choices of transit riders, but also can be used to improve the characterization of urban built form by uncovering the likely activity landscapes of various places. The proposed framework and the methodology is demonstrated by focusing on the use of transit smart card transaction data, i.e., EZ-Link data, to study activity-travel patterns in Singapore. Although different modules of the framework are loosely coupled at the moment, we have tried to pipeline as much of the process as possible to facilitate efficient data processing and analysis. This allows researchers and planners to keep track of the evolution of human activity-travel patterns over time, and examine the correlations between the changes in activities and the changes in the built environment. The knowledge gained from continuous urban sensing data will certainly help policy makers and planners understand the current states of urban dynamics and monitor changes as transportation infrastructure and travel behaviors evolve over time.
Description
Thesis: Ph. D. in Urban and Regional Planning, Massachusetts Institute of Technology, Department of Urban Studies and Planning, 2014. Cataloged from PDF version of thesis. Includes bibliographical references (pages 149-157).
Date issued
2014Department
Massachusetts Institute of Technology. Department of Urban Studies and PlanningPublisher
Massachusetts Institute of Technology
Keywords
Urban Studies and Planning.