Characterizing shale gas and tight oil drilling and production performance variability
Author(s)
Montgomery, Justin B. (Justin Bruce)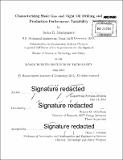
DownloadFull printable version (18.94Mb)
Other Contributors
Massachusetts Institute of Technology. Technology and Policy Program.
Advisor
Francis M. O'Sullivan.
Terms of use
Metadata
Show full item recordAbstract
Shale gas and tight oil are energy resources of growing importance to the U.S. and the world. The combination of horizontal drilling and hydraulic fracturing has enabled economically feasible production from these resources, leading to a surge in domestic oil and gas production. This is providing an economic boon and reducing reliance on foreign sources of energy in the U.S., but there are still a number of environmental, economic, and technical challenges that must be overcome to unlock the resource's full potential. One key challenge is understanding variability in individual well performance-in terms of both drilling time (a key driver of well cost) and well productivity-which has led to greater than anticipated economic risk associated with shale gas and tight oil development. Thus far, more reliable forecasting has remained elusive due to its prohibitive cost and the poorly understood nature of the resource. There is an opportunity to make use of available drilling and production data to improve the characterization of variability. For my analysis, I use publicly-available well production data and drilling reports from a development campaign. In order to characterize variability, I use a combination of graphical, statistical, and data analytics methods. For well productivity, I use probability plots to demonstrate a universality to the distribution shape, which can accurately be described as lognormal. Building on this distributional assumption, I demonstrate the utility of Bayesian statistical inference for improving estimates of the distribution parameters, which will allow companies to better anticipate resource variability and make better decisions under this uncertainty. For drilling, I characterize variability in operations by using approximate string matching to compare drilling activity sequences, leading to a metric for operational variability. Activity sequences become more similar over time, consistent with the notion of standardization. Finally, I investigate variability of drilling times as they progress along the learning curve, using probability plots again. I find some indication of lognormality, with implications for how learning in drilling should be measured and predicted. This thesis emphasizes the relevance of data analytics to characterizing performance variability across the spectrum in shale gas and tight oil. The findings also demonstrate the value of such an approach for identifying patterns of behavior, estimating future variability, and guiding development strategies.
Description
Thesis: S.M. in Technology and Policy, Massachusetts Institute of Technology, Engineering Systems Division, Technology and Policy Program, 2015. Cataloged from PDF version of thesis. Includes bibliographical references (pages 137-147).
Date issued
2015Department
Massachusetts Institute of Technology. Engineering Systems Division; Technology and Policy ProgramPublisher
Massachusetts Institute of Technology
Keywords
Engineering Systems Division., Technology and Policy Program.