Predicting and improving invoice-to-cash collection through machine learning/
Author(s)
Hu, Peiguang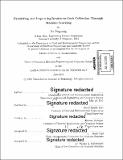
DownloadFull printable version (7.319Mb)
Alternative title
Massachusetts Institute of Technology. Department of Electrical Engineering and Computer Science.
Other Contributors
Massachusetts Institute of Technology. Department of Civil and Environmental Engineering.
Advisor
David Simchi-Levi and Asuman Ozdaglar.
Terms of use
Metadata
Show full item recordAbstract
Delinquent invoice payments can be a source of financial instability if it is poorly managed. Research in supply chain finance shows that effective invoice collection is positively correlated with the overall financial performance of companies. In this thesis I address the problem of predicting the delinquent invoice payments in advance with machine learning of historical invoice data. Specifically, this thesis demonstrates how supervised learning models can be used to detect the invoices that would have delay payments, as well as the problematic customers, which enables customized collection actions from the firm. The model from this thesis can predict with high accuracy if an invoice will be paid on time or not and also estimate the magnitude of the delay. This thesis builds and trains its invoice delinquency prediction capability based on the real-world invoice data from a Fortune 500 company.
Description
Thesis: S.M. in Transportation, Massachusetts Institute of Technology, Department of Civil and Environmental Engineering, 2015. Thesis: S.M., Massachusetts Institute of Technology, Department of Electrical Engineering and Computer Science, 2015. Cataloged from PDF version of thesis. Includes bibliographical references (pages 89-92).
Date issued
2015Department
Massachusetts Institute of Technology. Department of Civil and Environmental Engineering; Massachusetts Institute of Technology. Department of Electrical Engineering and Computer SciencePublisher
Massachusetts Institute of Technology
Keywords
Civil and Environmental Engineering.