Semidenite representations with applications in estimation and inference
Author(s)
Saunderson, James (James Francis)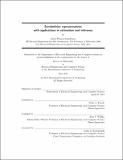
DownloadFull printable version (2.561Mb)
Other Contributors
Massachusetts Institute of Technology. Department of Electrical Engineering and Computer Science.
Advisor
Pablo A. Parrilo and Alan S. Willsky.
Terms of use
Metadata
Show full item recordAbstract
Semidenite optimization problems are an expressive family of convex optimization problems that can be solved eciently. We develop semidenite optimization-based formulations and approximations for a number of families of optimization problems, including problems arising in spacecraft attitude estimation and in learning tree-structured statistical models. We construct explicit exact reformulations of two families of optimization problems in terms of semidenite optimization. The first family are linear optimization problems over the derivative relaxations of spectrahedral cones. The second family are linear optimization problems over rotation matrices, i.e. orthogonal matrices with unit determinant. We use our semidenite description of linear optimization problems over rotation matrices to express a joint spin-rate and attitude estimation problem for a spinning spacecraft exactly as a semidenite optimization problem. For families of optimization problems that are, in general, intractable, one cannot hope for ecient semidenite optimization-based formulations. Nevertheless, there are natural ways to develop approximations for these problems called semidenite relaxations. We analyze one such relaxation of a broad family of optimization problems with multiple variables interacting pairwise, including, for instance, certain multivariate optimization problems over rotation matrices. We characterize the worst-case gap between the optimal value of the original problem and a particular semidenite relaxation, and develop systematic methods to round solutions of the semidenite relaxation to feasible points of the original problem. Our results establish a correspondence between the analysis of rounding schemes for these problems and a natural geometric optimization problem that we call the normalized maximum width problem. We also develop semidenite optimization-based methods for a statistical modeling problem. The problem involves realizing a given multivariate Gaussian distribution as the marginal distribution among a subset of variables in a Gaussian tree model. This is desirable because Gaussian tree models enjoy certain conditional independence relations that allow for very ecient inference. We reparameterize this realization problem as a structured matrix decomposition problem and show how it can be approached using a semidenite optimization formulation. We establish sucient conditions on the parameters and structure of an underlying Gaussian tree model so that our methods can recover it from the marginal distribution on its leaf-indexed variables.
Description
Thesis: Ph. D., Massachusetts Institute of Technology, Department of Electrical Engineering and Computer Science, 2015. This electronic version was submitted by the student author. The certified thesis is available in the Institute Archives and Special Collections. Cataloged from student-submitted PDF version of thesis. Includes bibliographical references (pages 235-244).
Date issued
2015Department
Massachusetts Institute of Technology. Department of Electrical Engineering and Computer SciencePublisher
Massachusetts Institute of Technology
Keywords
Electrical Engineering and Computer Science.