Spoken language understanding in a nutrition dialogue system
Author(s)
Korpusik, Mandy B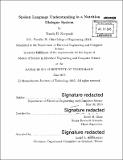
DownloadFull printable version (9.859Mb)
Other Contributors
Massachusetts Institute of Technology. Department of Electrical Engineering and Computer Science.
Advisor
James R. Glass.
Terms of use
Metadata
Show full item recordAbstract
Existing approaches for the prevention and treatment of obesity are hampered by the lack of accurate, low-burden methods for self-assessment of food intake, especially for hard-to-reach, low-literate populations. For this reason, we propose a novel approach to diet tracking that utilizes speech understanding and dialogue technology in order to enable efficient self-assessment of energy and nutrient consumption. We are interested in studying whether speech can lower user workload compared to existing self-assessment methods, whether spoken language descriptions of meals can accurately quantify caloric and nutrient absorption, and whether dialogue can efficiently and effectively be used to ascertain and clarify food properties, perhaps in conjunction with other modalities. In this thesis, we explore the core innovation of our nutrition system: the language understanding component which relies on machine learning methods to automatically detect food concepts in a user's spoken meal description. In particular, we investigate the performance of conditional random field (CRF) models for semantic labeling and segmentation of spoken meal descriptions. On a corpus of 10,000 meal descriptions, we achieve an average F1 test score of 90.7 for semantic tagging and 86.3 for associating foods with properties. In a study of users interacting with an initial prototype of the system, semantic tagging achieved an accuracy of 83%, which was sufficiently high to satisfy users.
Description
Thesis: S.M., Massachusetts Institute of Technology, Department of Electrical Engineering and Computer Science, 2015. Cataloged from PDF version of thesis. Includes bibliographical references (pages 105-111).
Date issued
2015Department
Massachusetts Institute of Technology. Department of Electrical Engineering and Computer SciencePublisher
Massachusetts Institute of Technology
Keywords
Electrical Engineering and Computer Science.