An automatic, multi-fidelity framework for optimizing the performance of super-cavitating hydrofoils using Gaussian process regression and Bayesian optimization
Author(s)
Parker, Benjamin W. (Benjamin Wade)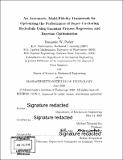
DownloadFull printable version (12.72Mb)
Other Contributors
Massachusetts Institute of Technology. Department of Mechanical Engineering.
Advisor
Michael Triantafyllou.
Terms of use
Metadata
Show full item recordAbstract
Computer automated design of complex physical systems is often limited by the computational resources required for the high precision solvers. Determining an optimum design necessitates high accuracy simulations due to the multi-dimensional design space and the interconnectedness of the constraint and objective quantities. This paper will present an automated framework for iterating through a design loop that includes both physics-based computer simulations and surrogate model training using machine learning techniques. To alleviate the computation burden and efficiently explore the design space, a surrogate model for each quantity of interest that cannot be found deterministically will be utilized. Further reduction of the computational cost is accomplished by utilizing both low- and high-fidelity data to build the response surfaces. These response surface models will be trained using multi-fidelity Gaussian process regression. The models will be iteratively improved using Bayesian optimization and additional high-fidelity simulations that are automatically initiated within the design loop. In addition, Bayesian optimization will be used to automatically determine the optimum kernel for the Gaussian regression model. The feasibility of this framework is demonstrated by designing a 2D super-cavitating hydrofoil and comparing the optimum shape found with a known benchmark design. This automated multi-fidelity Bayesian optimization framework can aid in taking the human out of the design loop, thus freeing manpower resources and removing potential human bias.
Description
Thesis: Nav. E., Massachusetts Institute of Technology, Department of Mechanical Engineering, 2018. Thesis: S.M., Massachusetts Institute of Technology, Department of Mechanical Engineering, 2018. Cataloged from PDF version of thesis. Includes bibliographical references (pages 99-100).
Date issued
2018Department
Massachusetts Institute of Technology. Department of Mechanical EngineeringPublisher
Massachusetts Institute of Technology
Keywords
Mechanical Engineering.