Analytical and simulation models for flexible, robotic automotive assembly lines
Author(s)
Tung, Yi-Shiuan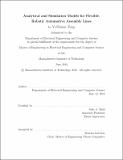
DownloadFull printable version (2.473Mb)
Other Contributors
Massachusetts Institute of Technology. Department of Electrical Engineering and Computer Science.
Advisor
Julie A. Shah.
Terms of use
Metadata
Show full item recordAbstract
To adapt to the rapidly changing market, the automotive industry is interested in flexible assembly lines that can handle disruptions due to machine failures or schedule changes. In this thesis, we propose a new assembly line layout (flexible layout) that uses mobile, robotic platforms to transport cars, which can move out of the line when disruptions occur to prevent blocking other cars on the line. We use discrete event simulation and analytical models to analyze the throughput of the new layout in a single band and two bands with a finite buffer. The simulation results show that the new layout achieves an average of 25.6% and 35.9% speed up over the conventional layout for the single band and two bands cases respectively. We improve previous two-machine line analytical models by augmenting the state of the Markov chain to model every machine in a band. We show that the augmented discrete Markov chain model predicts the throughput within 13% and 18% of the simulation throughput for the conventional and flexible layouts respectively. We further evaluate whether the flexible layout can improve its throughput by learning a policy for parking the platforms. By modeling the agents as independent learners, we apply single-agent reinforcement learning algorithms and show that the policy learned works well but suffers from lack of coordination.
Description
Thesis: M. Eng., Massachusetts Institute of Technology, Department of Electrical Engineering and Computer Science, 2018. This electronic version was submitted by the student author. The certified thesis is available in the Institute Archives and Special Collections. Cataloged from student-submitted PDF version of thesis. Includes bibliographical references (pages 117-120).
Date issued
2018Department
Massachusetts Institute of Technology. Department of Electrical Engineering and Computer SciencePublisher
Massachusetts Institute of Technology
Keywords
Electrical Engineering and Computer Science.