Large-margin Gaussian mixture modeling for automatic speech recognition
Author(s)
Chang, Hung-An, Ph. D. Massachusetts Institute of Technology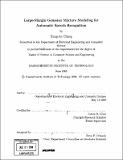
DownloadFull printable version (10.44Mb)
Other Contributors
Massachusetts Institute of Technology. Dept. of Electrical Engineering and Computer Science.
Advisor
James R. Glass.
Terms of use
Metadata
Show full item recordAbstract
Discriminative training for acoustic models has been widely studied to improve the performance of automatic speech recognition systems. To enhance the generalization ability of discriminatively trained models, a large-margin training framework has recently been proposed. This work investigates large-margin training in detail, integrates the training with more flexible classifier structures such as hierarchical classifiers and committee-based classifiers, and compares the performance of the proposed modeling scheme with existing discriminative methods such as minimum classification error (MCE) training. Experiments are performed on a standard phonetic classification task and a large vocabulary speech recognition (LVCSR) task. In the phonetic classification experiments, the proposed modeling scheme yields about 1.5% absolute error reduction over the current state of the art. In the LVCSR experiments on the MIT lecture corpus, the large-margin model has about 6.0% absolute word error rate reduction over the baseline model and about 0.6% absolute error rate reduction over the MCE model.
Description
Thesis (S.M.)--Massachusetts Institute of Technology, Dept. of Electrical Engineering and Computer Science, 2008. Includes bibliographical references (p. 101-103).
Date issued
2008Department
Massachusetts Institute of Technology. Department of Electrical Engineering and Computer SciencePublisher
Massachusetts Institute of Technology
Keywords
Electrical Engineering and Computer Science.