Supervised vs. Unsupervised Learning for Operator State Modeling in Unmanned Vehicle Settings
Author(s)
Boussemart, Y.; Cummings, M.L.; Las Fargeas, J.; Roy, N.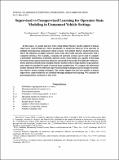
DownloadAIAA-46767-263.pdf (507.6Kb)
Metadata
Show full item recordAbstract
In this paper, we model operator states using hidden Markov models applied to human supervisory control behaviors. More specifically, we model the behavior of an operator of multiple heterogeneous unmanned vehicle systems. The hidden Markov model framework allows the inference of higher operator states from observable operator interaction with a computer interface. For example, a sequence of operator actions can be used to compute a probability distribution of possible operator states. Such models are capable of detecting deviations from expected operator behavior as learned by the model.The difficulty with parametric inference models such as hidden Markov models is that a large number of parameters must either be specified by hand or learned from example data.We compare the behavioral
models obtained with two different supervised learning techniques and an unsupervised hidden Markov model training technique. The results suggest that the best models of human supervisory control behavior are obtained through unsupervised learning. We conclude by presenting further extensions to this work.
Date issued
2011Publisher
Journal of Aerospace Computing, Information, and Communication
Citation
Boussemart, Y., Cummings, M.L., Las Fargeas, J., Roy, N., Supervised vs. Unsupervised Learning for Operator State Modeling in Unmanned Vehicle Settings, Journal of Aerospace Computing, Information, and Communication (1542-9423) vol. 8 no. 3, pg 71-85, 2011.
Other identifiers
DOI: 10.2514/1.46767