Optimal Bayesian experimental design in the presence of model error
Author(s)
Feng, Chi, S.M. Massachusetts Institute of Technology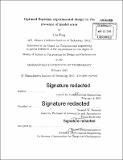
DownloadFull printable version (10.27Mb)
Other Contributors
Massachusetts Institute of Technology. Computation for Design and Optimization Program.
Advisor
Youssef M. Marzouk.
Terms of use
Metadata
Show full item recordAbstract
The optimal selection of experimental conditions is essential to maximizing the value of data for inference and prediction. We propose an information theoretic framework and algorithms for robust optimal experimental design with simulation-based models, with the goal of maximizing information gain in targeted subsets of model parameters, particularly in situations where experiments are costly. Our framework employs a Bayesian statistical setting, which naturally incorporates heterogeneous sources of information. An objective function reflects expected information gain from proposed experimental designs. Monte Carlo sampling is used to evaluate the expected information gain, and stochastic approximation algorithms make optimization feasible for computationally intensive and high-dimensional problems. A key aspect of our framework is the introduction of model calibration discrepancy terms that are used to "relax" the model so that proposed optimal experiments are more robust to model error or inadequacy. We illustrate the approach via several model problems and misspecification scenarios. In particular, we show how optimal designs are modified by allowing for model error, and we evaluate the performance of various designs by simulating "real-world" data from models not considered explicitly in the optimization objective.
Description
Thesis: S.M., Massachusetts Institute of Technology, Computation for Design and Optimization Program, 2015. Cataloged from PDF version of thesis. Includes bibliographical references (pages 87-90).
Date issued
2015Department
Massachusetts Institute of Technology. Computation for Design and Optimization ProgramPublisher
Massachusetts Institute of Technology
Keywords
Computation for Design and Optimization Program.