Latent source models for nonparametric inference
Author(s)
Chen, George H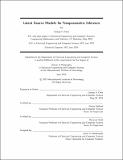
DownloadFull printable version (2.041Mb)
Other Contributors
Massachusetts Institute of Technology. Department of Electrical Engineering and Computer Science.
Advisor
Polina Golland and Devavrat Shah.
Terms of use
Metadata
Show full item recordAbstract
Nearest-neighbor inference methods have been widely and successfully used in numerous applications such as forecasting which news topics will go viral, recommending products to people in online stores, and delineating objects in images by looking at image patches. However, there is little theoretical understanding of when, why, and how well these nonparametric inference methods work in terms of key problem-specific quantities relevant to practitioners. This thesis bridges the gap between theory and practice for these methods in the three specific case studies of time series classification, online collaborative filtering, and patch-based image segmentation. To do so, for each of these problems, we prescribe a probabilistic model in which the data appear generated from unknown "latent sources" that capture salient structure in the problem. These latent source models naturally lead to nearest-neighbor or nearest-neighbor-like inference methods similar to ones already used in practice. We derive theoretical performance guarantees for these methods, relating inference quality to the amount of training data available and problems-specific structure modeled by the latent sources.
Description
Thesis: Ph. D., Massachusetts Institute of Technology, Department of Electrical Engineering and Computer Science, 2015. This electronic version was submitted by the student author. The certified thesis is available in the Institute Archives and Special Collections. Cataloged from student-submitted PDF version of thesis. Includes bibliographical references (pages 95-101).
Date issued
2015Department
Massachusetts Institute of Technology. Department of Electrical Engineering and Computer SciencePublisher
Massachusetts Institute of Technology
Keywords
Electrical Engineering and Computer Science.