Multiple model estimation for linear stochastic hybrid systems with non-homogeneous transition probabilities
Author(s)
Kasperski, Michael William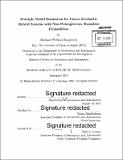
DownloadFull printable version (3.616Mb)
Other Contributors
Massachusetts Institute of Technology. Department of Aeronautics and Astronautics.
Advisor
Hamsa Balakrishnan.
Terms of use
Metadata
Show full item recordAbstract
This thesis investigates the field of stochastic hybrid estimation. A broad introduction to the framework surrounding estimation, filtering, and multiple model based systems is presented. More specifically, the often made assumption of a constant time-invariant mode transition probability matrix is relaxed. Recent work done in the area of non-Markov jump stochastic hybrid systems is explored, including semi- Markov systems, non-homogeneous transition probability matrices, and continuous-state-dependent mode transitions. Algorithms needed to develop linear multiple model based filters with non-homogeneous transition probabilities are detailed. Finally, a case study for the practical implementation of an extended Kalman filter in the application of attitude heading and reference systems is conducted.
Description
Thesis: S.M., Massachusetts Institute of Technology, Department of Aeronautics and Astronautics, 2015. Cataloged from PDF version of thesis. Includes bibliographical references (pages 61-64).
Date issued
2015Department
Massachusetts Institute of Technology. Department of Aeronautics and AstronauticsPublisher
Massachusetts Institute of Technology
Keywords
Aeronautics and Astronautics.