Bayesian computational models for inferring preferences
Author(s)
Evans, Owain Rhys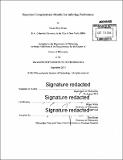
DownloadFull printable version (14.58Mb)
Other Contributors
Massachusetts Institute of Technology. Department of Linguistics and Philosophy.
Advisor
Roger White.
Terms of use
Metadata
Show full item recordAbstract
This thesis is about learning the preferences of humans from observations of their choices. It builds on work in economics and decision theory (e.g. utility theory, revealed preference, utilities over bundles), Machine Learning (inverse reinforcement learning), and cognitive science (theory of mind and inverse planning). Chapter 1 lays the conceptual groundwork for the thesis and introduces key challenges for learning preferences that motivate chapters 2 and 3. I adopt a technical definition of 'preference' that is appropriate for inferring preferences from choices. I consider what class of objects preferences should be defined over. I discuss the distinction between actual preferences and informed preferences and the distinction between basic/intrinsic and derived/instrumental preferences. Chapter 2 focuses on the challenge of human 'suboptimality'. A person's choices are a function of their beliefs and plans, as well as their preferences. If they have inaccurate beliefs or make inefficient plans, then it will generally be more difficult to infer their preferences from choices. It is also more difficult if some of their beliefs might be inaccurate and some of their plans might be inefficient. I develop models for learning the preferences of agents subject to false beliefs and to time inconsistency. I use probabilistic programming to provide a concise, extendable implementation of preference inference for suboptimal agents. Agents performing suboptimal sequential planning are represented as functional programs. Chapter 3 considers how preferences vary under different combinations (or &compositions') of outcomes. I use simple mathematical functional forms to model composition. These forms are standard in microeconomics, where the outcomes in question are quantities of goods or services. These goods may provide the same purpose (and be substitutes for one another). Alternatively, they may combine together to perform some useful function (as with complements). I implement Bayesian inference for learning the preferences of agents making choices between different combinations of goods. I compare this procedure to empirical data for two different applications.
Description
Thesis: Ph. D. in Linguistics, Massachusetts Institute of Technology, Department of Linguistics and Philosophy, 2015. Cataloged from PDF version of thesis. Includes bibliographical references (pages 130-131).
Date issued
2015Department
Massachusetts Institute of Technology. Department of Linguistics and PhilosophyPublisher
Massachusetts Institute of Technology
Keywords
Linguistics and Philosophy.