Model order reduction for stochastic models of biomolecular systems with time-scale separation
Author(s)
Herath, Narmada Kumari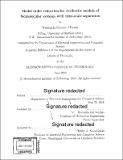
DownloadFull printable version (13.90Mb)
Other Contributors
Massachusetts Institute of Technology. Department of Electrical Engineering and Computer Science.
Advisor
Domitilla Del Vecchio.
Terms of use
Metadata
Show full item recordAbstract
Biomolecular systems often involve reactions that take place on different time-scales, giving rise to 'slow' and 'fast' system variables. This property is widely used in the analysis of systems to obtain dynamical models with reduced dimensions. In deterministic systems, methods to obtain such reduced-order models are well defined by the singular perturbation or averaging techniques. However, model reduction of stochastic systems remains an ongoing area of research. In particular, existing model reduction methods for stochastic models of biomolecular systems lack rigorous error quantifications between the full and reduced dynamics. Furthermore, they only provide approximations for the slow variable dynamics, making the application of such methods to biomolecular systems difficult since the variables of interest are typically mixed (i.e., they encompass both fast and slow variables). In this thesis, we consider biomolecular systems modeled using the chemical Langevin equation (CLE) and the Linear Noise Approximation (LNA). Specifically, we consider biomolecular systems with linear propensity functions modeled by the CLE and systems with arbitrary propensity functions modeled by the LNA. For these systems, we obtain reduced-order models that approximate both the slow and fast variables under time-scale separation conditions. In particular, with suitable assumptions, we prove that the moments of the reduced-order models converge to those of the full systems as the time-scale separation becomes large. Our results further provide a rigorous justification for the accuracy of the stochastic total quasi-steady state approximation (tQSSA). We then consider two applications of these reduced-order models. In the first application, we analyze the trade-offs between modularity and signal noise in biomolecular networks. In the second application, we consider the application of the reduced-order LNA developed in this work to obtain reduced-order stochastic models for gene-regulatory networks.
Description
Thesis: Ph. D., Massachusetts Institute of Technology, Department of Electrical Engineering and Computer Science, 2018. Cataloged from PDF version of thesis. Includes bibliographical references (pages 177-183).
Date issued
2018Department
Massachusetts Institute of Technology. Department of Electrical Engineering and Computer SciencePublisher
Massachusetts Institute of Technology
Keywords
Electrical Engineering and Computer Science.