Machine learning non-local closures for turbulent anisotropic multiphase flows
Author(s)
Charalampopoulos, Alexis-Tzianni.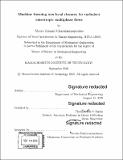
Download1138990951-MIT.pdf (6.305Mb)
Other Contributors
Massachusetts Institute of Technology. Department of Mechanical Engineering.
Advisor
Themistoklis P. Sapsis.
Terms of use
Metadata
Show full item recordAbstract
In this thesis we formulate a data-driven, nonlocal closure scheme for turbulent multiphase fluid flows. In more detail, we use the predictions of neural nets to actively integrate in time the evolution of a turbulent anisotropic fluid flow on which bubbles that act as passive inertial tracers are being transported. The first step of our method requires the introduction of a filter on the initial 2D dynamical system that reduces it to a 1D problem. Then we model the appearing closure terms using recurrent neural networks and convolutions in space. We avoid the use of fully-connected layers due to their large computational overhead, tendency to over-fit on training data and nonphysical implications. We choose to work with recurrent neural networks as recent works have shown memory effects can enhance data-driven predictions in applications in turbulence. We first test our method on unimodal jets and then proceed to bimodal profiles. Our model appears to accurately learn the statistical steady state profile of both the fluid flow velocity field as well as the profile of the distribution of bubbles in space. Finally, we use neural networks to learn the statistics of bubble deformation so that we can incorporate the effects of the bubble motion back to the fluid flow itself, on a later stage.
Description
Thesis: S.M., Massachusetts Institute of Technology, Department of Mechanical Engineering, 2019 Cataloged from PDF version of thesis. Includes bibliographical references (pages 65-68).
Date issued
2019Department
Massachusetts Institute of Technology. Department of Mechanical EngineeringPublisher
Massachusetts Institute of Technology
Keywords
Mechanical Engineering.