Deep embedding approach to classify purpose of trips between cities from GPS data
Author(s)
Alhazzani, May,S.M.Massachusetts Institute of Technology.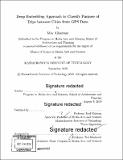
Download1142235684-MIT.pdf (9.042Mb)
Other Contributors
Program in Media Arts and Sciences (Massachusetts Institute of Technology)
Advisor
Iyad Rahwan.
Terms of use
Metadata
Show full item recordAbstract
I present a computational framework to identify purpose of trips between cities from GPS traces using a deep embedding approach. I extracted statistical features that captures trips characteristics that includes: temporal features, spatial features and Points of Interests (POI) features. I deployed a deep learning model to extract representative features in a lower dimensional space, which I then feed to a classic clustering algorithm to uncover purpose of trips. I detected six main purposes from trips coming from five different metropolitan areas in the United States to New York city. The trips' purposes detected are: work, which is the most dominating in size, entertainment, shopping, academic, and travelling. I interpret and discuss each cluster in terms of its features. I also compare cities from which trips originated by the distribution of their trips purposes.
Description
Thesis: S.M., Massachusetts Institute of Technology, School of Architecture and Planning, Program in Media Arts and Sciences, 2019 Cataloged from PDF version of thesis. Includes bibliographical references (pages 64-67).
Date issued
2019Department
Program in Media Arts and Sciences (Massachusetts Institute of Technology)Publisher
Massachusetts Institute of Technology
Keywords
Program in Media Arts and Sciences