A Forecasting Face-Off for Oil and Gas Spare Parts
Author(s)
Serry, Mahmood; Vasa, James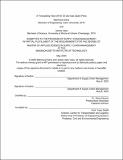
DownloadFull capstone (1.532Mb)
Metadata
Show full item recordAbstract
Spare parts demand forecasting is a key activity for asset intensive industries, but it is challenging due to the underlying demand characteristics. Demand is characterized by periods of zero demand arrivals; and the size of the order is variable with large, unexpected spikes. Schlumberger, an oil and gas service manufacturer, is facing the issue of low forecast accuracy for its spare parts, and has challenged the team to improve it. This research uses machine learning techniques to improve demand forecasting accuracy of spare parts for Schlumberger. The methodology of the research starts with classifying the parts into four classes namely: smooth; intermittent; erratic; and lumpy. Then, we apply recommended time series based on the literature for forecasting four classes. The time series forecast was then fed as features along with judgmental forecast and the demand parameters into two different machine learning algorithms, namely Classification and Regression Trees (CART) and Random Forests. Both models showed more than 75% improvement in accuracy over conventional demand forecasting methods when measured by Root Mean Squared Error. This improvement shows the potential benefit of adding human judgement as a parameter into machine learning algorithms when forecasting spare parts.
Date issued
2020-08-06Keywords
demand planning, machine learning