Hardware-efficient deep learning for 3D point cloud
Author(s)
Liu, Zhijian,S. M.Massachusetts Institute of Technology.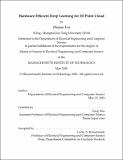
Download1192486856-MIT.pdf (13.02Mb)
Other Contributors
Massachusetts Institute of Technology. Department of Electrical Engineering and Computer Science.
Advisor
Song Han.
Terms of use
Metadata
Show full item recordAbstract
3D neural networks are widely used in real-world applications (e.g., AR/VR headsets, self-driving cars). They are required to be fast and accurate; however, limited hardware resources on edge devices make these requirements rather challenging. Previous work processes 3D data using either voxel-based or point-based neural networks; while both types of 3D models are not hardware-efficient because of the large memory footprint and random memory access. In this thesis, we study 3D deep learning from the efficiency perspective. We first systematically analyze the bottlenecks of previous 3D methods. We then combine the best from point-based and voxel-based models together and propose a novel hardware-efficient 3D primitive, Point-Voxel Convolution (PVConv). We further enhance this primitive with Sparse Convolution to make it more effective in processing large (outdoor) scenes. Based on our designed 3D primitive, we introduce 3D-NAS, a neural architecture search framework that is specialized for 3D, to explore the best network architecture given a resource constraint. We evaluate our proposed method on various tasks including 3D part segmentation (for objects), 3D semantic segmentation (for indoor and outdoor scenes), and 3D object detection (for outdoor scenes). Across all four benchmarks, our proposed method achieves the state-of-the-art performance with 2.8 measured speedup on average. Furthermore, our model has been deployed to the autonomous racing vehicle of MIT Driverless, achieving larger detection range, higher accuracy and lower latency for efficient LiDAR perception.
Description
Thesis: S.M., Massachusetts Institute of Technology, Department of Electrical Engineering and Computer Science, May, 2020 Cataloged from the official PDF of thesis. Includes bibliographical references (pages 59-64).
Date issued
2020Department
Massachusetts Institute of Technology. Department of Electrical Engineering and Computer SciencePublisher
Massachusetts Institute of Technology
Keywords
Electrical Engineering and Computer Science.