An empirical study identifying bias in Yelp dataset
Author(s)
Choi, Seri,M. Eng.Massachusetts Institute of Technology.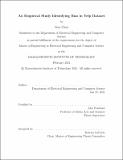
Download1251779073-MIT.pdf (2.873Mb)
Other Contributors
Massachusetts Institute of Technology. Department of Electrical Engineering and Computer Science.
Advisor
Alex Pentland.
Terms of use
Metadata
Show full item recordAbstract
Online review platforms have become an essential element of the business industry, providing users in-depth information on businesses and other users' experiences. The purpose of this study is to examine possible bias or discriminatory behaviors in users' rating habits in the Yelp dataset. The Surprise recommender system is utilized to produce expected ratings for the test set, training the model with 75% of the original dataset to learn the rating trends. Then, the ordinary least squares (OLS) linear regression is applied to identify which factors affected the percent change and which categories or locations show more bias than the others. This paper can provide insights into ways that bias can manifest within a dataset due to non-experimental factors such as social psychology; future research into this topic can therefore take these non-experimental factors, such as the discriminatory bias found in Yelp reviews, into consideration in order to reduce bias when utilizing machine learning algorithms.
Description
Thesis: M. Eng., Massachusetts Institute of Technology, Department of Electrical Engineering and Computer Science, February, 2021 Cataloged from the official PDF of thesis. Includes bibliographical references (pages 45-47).
Date issued
2021Department
Massachusetts Institute of Technology. Department of Electrical Engineering and Computer SciencePublisher
Massachusetts Institute of Technology
Keywords
Electrical Engineering and Computer Science.