Machine Learning for Downstream Oil & Gas Refineries: Applications for Solvent Deasphalting
Author(s)
Dowell, Christian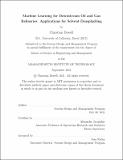
DownloadThesis PDF (13.30Mb)
Advisor
Jacquillat, Alexandre
Terms of use
Metadata
Show full item recordAbstract
This thesis seeks to provide continuous DAO yield estimations for an SDA unit by constructing modern machine learning models using data sets from a commercial downstream oil and gas refinery in the United States. These data sets include plant operating parameters and laboratory measurements for feed properties. The best machine learning model, determined via an extensive cross-validation procedure, exhibits high out-of-sample R^2 values of 0.76. Furthermore, this predictive machine learning model is incorporated into a linear optimization framework to enhance crude oil purchasing decisions for a downstream refinery. Results suggest that the proposed approach, combining predictive and prescriptive analytics, can result in significant profitability gains estimated at $730,000 annually. The results of this model can be utilized for more accurate plant monitoring within oil & gas downstream refineries, as well as improved decision making by oil and gas planning professionals.
Date issued
2021-09Department
System Design and Management Program.; System Design and Management Program.Publisher
Massachusetts Institute of Technology