Patterns of Moments Reasoning about Space Video via Pattern Language of Human Behavior by Extracting MultiAction Activities via Machine Learning Video
Author(s)
Wu, Ngai Hang (Charles)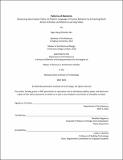
DownloadThesis PDF (20.79Mb)
Advisor
Nagakura, Takehiko
Terms of use
Metadata
Show full item recordAbstract
Architecture shapes our perception of space through scale, material, shape, and structure. The design of these elements convinces us of certain behaviors within and around the space, and it plays a significant role in our everyday lives. Experience oriented spatial design provides support for sustainable development, and improves people's material and physical satisfaction, well-being, and overall quality of life. It is contemptuous to think of architecture as a mere visual subject, but rather a medium where purposeful design of stimulus can be set up to lead to specific social behaviors in humans.
This thesis investigates the relationship between the built environment and human behavior through a data-driven method using on-location videos and machine learning. It is intended to provide a crucial means to understand the future opportunities that lie within responsive architecture and human-centered design. Human-centered design is conventionally a top-down approach that is highly dependent on architects’ subjective pedagogy and experience of a specific space and their dwellers’ and passengers' immediate needs. For example, Christopher Alexander published a collection of design patterns that promotes everyday users to become consciously aware of their living patterns around specific architectural setups. However, his prescriptive proposal outlines only his empirical insight, without further exploration into the dimension of culture, community, and time. The ability to understand human activities more thoroughly in space is lacking.
The research method is to observe and quantify human events and the types of spaces accommodating them and compare the behavioral difference within various spatial settings through short video clips. Initially, field data is collected by observing and recording human behaviors in public. Data-driven Computer Vision techniques are adopted, such as event recognition, scene attribute extraction, and dynamic analysis. Low-level features of human actions such as typing, drinking, stirring, and chewing are recognized, as well as the features of the surrounding space such as greenery, traffic, and enclosure. These low-level understandings discover behavioral patterns in different spaces with various features, providing insights into high-level human-centered spatial design.
After tests and analysis of a case conducted on street café designs, certain correlations between the properties of built environments and user behaviors were discovered. This case study demonstrated the adequacy of the proposed methodology to understand human behavior in space with the help of data-driven machine learning models. It can potentially be used to build a computational human-centered design system that designs by experience. For instance, such a system can help refitting a residential space to better-fit home office for work during pandemic situations.
Date issued
2022-05Department
Massachusetts Institute of Technology. Department of ArchitecturePublisher
Massachusetts Institute of Technology