Modeling and Evaluating Human Sound Localization in the Natural Environment
Author(s)
Francl, Andrew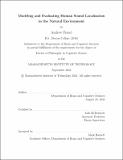
DownloadThesis PDF (13.03Mb)
Advisor
McDermott, Josh
Terms of use
Metadata
Show full item recordAbstract
Humans locate sounds in their environment to avoid danger and identify objects of interest. In a ten-minute bike ride, a person might take note of a car approaching from behind, a tree where a bird is singing, and pedestrians walking from around a blind corner.
Research on human sound localization has greatly advanced our understanding of binaural hearing but leaves us some ways from a complete understanding. In particular, it has been difficult to assess human sound localization in ways that align with humans experience on an everyday basis. This thesis aims to more closely align research methods and modeling approaches with the natural sound localization tasks that humans perform in the real world.
In the first study, we show that a model trained to localize sounds in naturalistic conditions exhibits many features of human spatial hearing. But when trained in unnatural environments without reverberation, noise, or natural sounds, the model’s performance characteristics deviate from those of humans. The results show how biological hearing is adapted to the challenges of real-world environments and illustrate how artificial neural networks can reveal the real-world constraints that shape perception.
In the second study, we ran a behavioral experiment to evaluate human sound localization in a naturalistic setting with natural sounds and identified specific sounds that are difficult for humans to localize. We assessed whether the model of sound localization from the first study could predict the accuracy with which individual sounds are localized. We found that the model predicted human localization accuracy well above chance. However, the model biases were distinct from those evident in humans, suggesting room for future improvement.
In the third study, we constructed a model that uses a biologically inspired learning approach to localizing sounds, relying on self-motion cues from head movements to learn representations of sound locations. We show that this strategy can learn a representation that enables accurate decoding of sound location without having access to the ground truth location for sounds during training.
In the fourth study, we used a model of human speech perception as a perceptual metric to improve speech denoising. We found that while this perceptual metric improved denoising over standard approaches, a simple model of the cochlea performed similarly, suggesting much of the benefit of this approach may be in using a frequency-based overcomplete representation of the signal.
Date issued
2022-09Department
Massachusetts Institute of Technology. Department of Brain and Cognitive SciencesPublisher
Massachusetts Institute of Technology