Learning Algorithms for Mixtures of Linear Dynamical Systems: A Practical Approach
Author(s)
Kumar, Nitin A.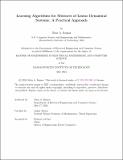
DownloadThesis PDF (708.4Kb)
Advisor
Moitra, Ankur
Terms of use
Metadata
Show full item recordAbstract
In this work, we give the first implementation of an algorithm to learn a mixture of linear dynamical systems (LDS’s), and an analysis of algorithms to learn a single linear dynamical system. Following the work of Bakshi et al. ([1]), we implement a recent polynomial-time algorithm based on a tensor decomposition with learning guarantees in a general setting, with some simplifications and minor optimizations. Our largest contribution is giving the first expectation-maximization (E-M) algorithm for learning a mixture of LDS’s, and an experimental evaluation against the Tensor Decomposition algorithm. We find that the E-M algorithm performs extremely well, and much better than the Tensor Decomposition algorithm. We analyze performance of these and other algorithms to learn both a single LDS and a mixture of LDS’s under various conditions (such as how much noise is present) and algorithm settings.
Date issued
2024-05Department
Massachusetts Institute of Technology. Department of Electrical Engineering and Computer SciencePublisher
Massachusetts Institute of Technology