fMRI detection with spatial regularization
Author(s)
Ou, Wanmei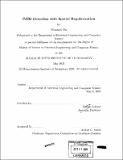
DownloadFull printable version (7.707Mb)
Alternative title
Functional Magnetic Resonant Imaging detection with spatial regularization
Other Contributors
Massachusetts Institute of Technology. Dept. of Electrical Engineering and Computer Science.
Advisor
Polina Golland.
Terms of use
Metadata
Show full item recordAbstract
Functional Magnetic Resonant Imaging (fMRI) is a non-invasive imaging technique used to study the brain. Neuroscientists have developed various algorithms to determine which voxels of the images are active. Most of these algorithms, operating on the signal of each voxel separately, are referred to as the voxel-by-voxel detectors. Among those voxel-by-voxel detectors, paired T-test and General Linear Model (GLM) are the most popular. The Mutual Information (MI) based detector has recently been introduced. It is interesting to compare these three detectors' modeling assumptions, as well as their performance, in order to understand their advantages and shortcomings. Due to the low signal-to-noise ratio (SNR), the voxel-by-voxel detectors usually result in fragmented activation pattern, which is not in agreement with our understanding of brain activation. The biological models of brain activation suggest that adjacent locations of the brain tend to be in the same activation state. We take advantage of these models and apply a Markov Random Field (MRF) spatial prior to the statistics provided by the voxel-by-voxel algorithms. MRF has been shown to be able to overcome the effect of over-smoothing, which is the major drawback of the conventional spatial regularization models such as the Gaussian smoothing model. (cont.) We adopt Mean Field, a variational algorithm, to estimate the MRF solution. We show that Mean Field can provide reasonable approximation compared with the exact solver in the case of binary MRFs, while reducing the computations by one to two orders of magnitude in our simulated and real data sets. In addition, unlike the exact solver, it can handle multiple-state MRFs. Inspired by atlas-based segmentation, we further refine the spatial regularization process by incorporating anatomical information, such as segmentation results from Magnetic Resonance Imaging (MRI), into the MRF prior. The extended MRF model encodes both tissue type and activation state. To our knowledge, our approach is the first spatial smoothing method that utilizes anatomical information without cortical surface extraction. To evaluate the smoothing models, we performed ROC and confusion matrix analysis on synthetic data. We also evaluate them by studying their ability to recover activation from significantly shorter time course using real data. Including anatomical information improves detection accuracy for both the Gaussian-smoothing-based detector and the MRF-based detector. The Gaussian-smoothing model provides poor results if we are interested in both positive and negative activation regions in the brain. (cont.) Furthermore, the anatomically guided MRF-based detector improves the detection quality compared with the anatomically guided Gaussian-smoothing-based detector for standard fMRI in standard SNR quality.
Description
Thesis (S.M.)--Massachusetts Institute of Technology, Dept. of Electrical Engineering and Computer Science, 2005. Includes bibliographical references (p. 99-102).
Date issued
2005Department
Massachusetts Institute of Technology. Department of Electrical Engineering and Computer SciencePublisher
Massachusetts Institute of Technology
Keywords
Electrical Engineering and Computer Science.