Whole genome regulatory variant evaluation for transcription factor binding
Author(s)
Zeng, Haoyang, Ph.D. Massachusetts Institute of Technology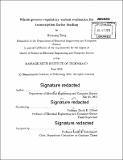
DownloadFull printable version (3.735Mb)
Alternative title
Whole genome WAVE for TF binding
Other Contributors
Massachusetts Institute of Technology. Department of Electrical Engineering and Computer Science.
Advisor
David K. Gifford.
Terms of use
Metadata
Show full item recordAbstract
With the advent of high-throughput sequencing technology, Genome Wide Association Studies (GWAS) have identified thousands of genetic variants that are associated with disease and complex traits. Many of these variants reside in the non-coding region of the genome, and affect gene expression and downstream cellular phenotype by disrupting the regulatory machinery of the cell. For example these variants can alter the binding of the transcription factors (TF). In this thesis we present Whole-genome regulAtory Variant Evaluation (WAVE), a computational method that models the TF binding ChIP-seq signal solely from DNA sequence and predicts genetic a variant's effect on TF binding. Applying WAVE to two important transcription factors, NFnB and CTCF, we show that WAVE accurately predicts ChIP-seq signal on held-out chromosome. WAVE discovers the DNA motif of the target TF as well as the binding co-factors, displaying substantially greater expressiveness in modeling TF binding than conventional motif-based approaches. Furthermore, with AUC larger than 0.7 in the most stringent control scenario, WAVE outperformed existing motif-based approaches in predicting genetic variants associated with allele-specific binding.
Description
Thesis: S.M., Massachusetts Institute of Technology, Department of Electrical Engineering and Computer Science, 2015. Cataloged from PDF version of thesis. Includes bibliographical references (pages 41-44).
Date issued
2015Department
Massachusetts Institute of Technology. Department of Electrical Engineering and Computer SciencePublisher
Massachusetts Institute of Technology
Keywords
Electrical Engineering and Computer Science.