A unified iteration space transformation framework for sparse and dense tensor algebra
Author(s)
Senanayake, Ryan Michael.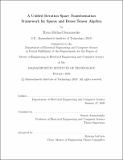
Download1237564498-MIT.pdf (2.424Mb)
Other Contributors
Massachusetts Institute of Technology. Department of Electrical Engineering and Computer Science.
Advisor
Saman Amarasinghe.
Terms of use
Metadata
Show full item recordAbstract
This work addresses the problem of optimizing mixed sparse and dense tensor algebra in a compiler. I show that standard loop transformations, such as strip-mining, tiling, collapsing, parallelization and vectorization, can be applied to irregular loops over sparse iteration spaces. I also show how these transformations can be applied to the contiguous value arrays of sparse tensor data structures, which I call their position spaces, to unlock load-balanced tiling and parallelism. These concepts have been prototyped in the open-source TACO system, where they are exposed as a scheduling API similar to the Halide domain-specific language for dense computations. Using this scheduling API, I show how to optimize mixed sparse/dense tensor algebra expressions, how to generate load-balanced code by scheduling sparse tensor algebra in position space, and how to generate sparse tensor algebra GPU code. As shown in the evaluation, these transformations allow us to generate code that is competitive with many hand-optimized implementations from the literature.
Description
Thesis: M. Eng., Massachusetts Institute of Technology, Department of Electrical Engineering and Computer Science, February, 2020 Cataloged from student-submitted PDF of thesis. Includes bibliographical references (pages 95-99).
Date issued
2020Department
Massachusetts Institute of Technology. Department of Electrical Engineering and Computer SciencePublisher
Massachusetts Institute of Technology
Keywords
Electrical Engineering and Computer Science.