Learning Semantic Scene Models by Trajectory Analysis
Author(s)
Wang, Xiaogang; Tieu, Kinh; Grimson, Eric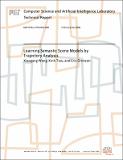
DownloadMIT-CSAIL-TR-2006-008.ps (17.46Mb)
Additional downloads
Other Contributors
Vision
Advisor
Eric Grimson
Metadata
Show full item recordAbstract
In this paper, we describe an unsupervised learning framework to segment a scene into semantic regions and to build semantic scene models from long-term observations of moving objects in the scene. First, we introduce two novel similarity measures for comparing trajectories in far-field visual surveillance. The measures simultaneously compare the spatial distribution of trajectories and other attributes, such as velocity and object size, along the trajectories. They also pro-vide a comparison confidence measure which indicates how well the measured im-age-based similarity approximates true physical similarity. We also introduce novel clustering algorithms which use both similarity and comparison confidence. Based on the proposed similarity measures and clustering methods, a framework to learn semantic scene models by trajectory analysis is developed. Trajectories are first clustered into vehicles and pedestrians, and then further grouped based on spatial and velocity distributions. Different trajectory clusters represent different activities. The geometric and statistical models of structures in the scene, such as roads, walk paths, sources and sinks, are automatically learned from the trajectory clusters. Abnormal activities are detected using the semantic scene models. The system is robust to low-level tracking errors.
Date issued
2006-02-10Other identifiers
MIT-CSAIL-TR-2006-008
Series/Report no.
Massachusetts Institute of Technology Computer Science and Artificial Intelligence Laboratory