Hydrologic data assimilation of multi-resolution microwave radiometer and radar measurements using ensemble smoothing
Author(s)
Dunne, Susan Catherine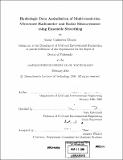
DownloadFull printable version (23.75Mb)
Other Contributors
Massachusetts Institute of Technology. Dept. of Civil and Environmental Engineering.
Advisor
Dara Entekhabi.
Terms of use
Metadata
Show full item recordAbstract
Previously, the ensemble Kalman filter (EnKF) has been used to estimate soil moisture and related fluxes by merging noisy low frequency microwave observations with forecasts from a conventional though uncertain land surface model (LSM). Here it is argued that soil moisture estimation is a reanalysis-type problem and thus smoothingis more appropriate than filtering. An ensemble moving batch smoother, an extension of the EnKF in which the state vector is distributed in time, is used to merge synthetic ESTAR observations with modeled soil moisture. Results demonstrate that smoothing can improve over filtering. However, augmentation of the state vector increases the computational cost significantly, rendering this approach unsuitable for spatially distributed problems. The ensemble Kalman smoother (EnKS) is an inexpensive alternative as the costly computations are already performed in the EnKF which provides the initial guess. It is used to assimilate observed L-band radiobrightness temperatures during the Southern Great Plains Experiment 1997. Estimated surface and root zone soil moisture is evaluated using gravimetric measurements and flux tower observations. It is shown that the EnKS can be implemented as a fixed-lag smoother with the required lag determined by the memory in subsurface soil moisture. In a synthetic experiment over the Arkansas-Red river basin, "true" soil moisture from the TOPLATS model is used to generate synthetic Hydros observations which are subsequently merged with modeled soil moisture from the Noah LSM using the EnKS. (cont.) It is shown that the EnKS can be used in a large problem, with a spatially distributed state vector, and spatially-distributed multi-resolution observations. This EnKS-based framework is used to study the synergy between passive and active observations, which have different resolutions and error distributions.
Description
Thesis (Ph. D.)--Massachusetts Institute of Technology, Dept. of Civil and Environmental Engineering, 2006. Includes bibliographical references (leaves 197-208).
Date issued
2006Department
Massachusetts Institute of Technology. Department of Civil and Environmental EngineeringPublisher
Massachusetts Institute of Technology
Keywords
Civil and Environmental Engineering.