Off-line calibration of Dynamic Traffic Assignment models
Author(s)
Balakrishna, Ramachandran, 1978-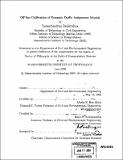
DownloadFull printable version (13.57Mb)
Alternative title
Off-line calibration of DTA models
Other Contributors
Massachusetts Institute of Technology. Dept. of Civil and Environmental Engineering.
Advisor
Moshe E. Ben-Akiva and Haris N. Koutsopoulos.
Terms of use
Metadata
Show full item recordAbstract
Advances in Intelligent Transportation Systems (ITS) have resulted in the deployment of surveillance systems that automatically collect and store extensive network-wide traffic data. Dynamic Traffic Assignment (DTA) models have also been developed for a variety of dynamic traffic management applications. Such models are designed to estimate and predict the evolution of congestion through detailed models and algorithms that capture travel demand, network supply and their complex interactions. The availability of rich time-varying traffic data spanning multiple days thus provides the opportunity to calibrate a DTA model's many inputs and parameters, so that its outputs reflect field conditions. The current state of the art of DTA model calibration is a sequential approach, in which supply model calibration (assuming known demand inputs) is followed by demand calibration with fixed supply parameters. In this thesis, we develop an off-line DTA model calibration methodology for the simultaneous estimation of all demand and supply inputs and parameters, using sensor data. We adopt a minimization formulation that can use any general traffic data, and present approaches to solve the complex, non-linear, stochastic optimization problem. (cont.) Case studies with DynaMIT, a DTA model with traffic estimation and prediction capabilities, are used to demonstrate and validate the proposed methodology. A synthetic traffic network with known demand parameters and simulated sensor data is used to illustrate the improvement over the sequential approach, the ability to accurately recover underlying model parameters, and robustness in a variety of demand and supply situations. Archived sensor data and a network from Los Angeles, CA are then used to demonstrate scalability. The benefit of the proposed methodology is validated through a real-time test of the calibrated DynaMIT's estimation and prediction accuracy, based on sensor data not used for calibration. Results indicate that the simultaneous approach significantly outperforms the sequential state of the art.
Description
Thesis (Ph. D.)--Massachusetts Institute of Technology, Dept. of Civil and Environmental Engineering, 2006. Includes bibliographical references (p. 197-212).
Date issued
2006Department
Massachusetts Institute of Technology. Department of Civil and Environmental EngineeringPublisher
Massachusetts Institute of Technology
Keywords
Civil and Environmental Engineering.