Learning to transform time series with a few examples
Author(s)
Rahimi, Ali, 1976-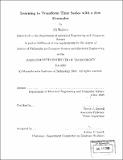
DownloadFull printable version (16.14Mb)
Other Contributors
Massachusetts Institute of Technology. Dept. of Electrical Engineering and Computer Science.
Advisor
Trevor J. Darrell.
Terms of use
Metadata
Show full item recordAbstract
I describe a semi-supervised regression algorithm that learns to transform one time series into another time series given examples of the transformation. I apply this algorithm to tracking, where one transforms a time series of observations from sensors to a time series describing the pose of a target. Instead of defining and implementing such transformations for each tracking task separately, I suggest learning a memoryless transformations of time series from a few example input-output mappings. The algorithm searches for a smooth function that fits the training examples and, when applied to the input time series, produces a time series that evolves according to assumed dynamics. The learning procedure is fast and lends itself to a closed-form solution. I relate this algorithm and its unsupervised extension to nonlinear system identification and manifold learning techniques. I demonstrate it on the tasks of tracking RFID tags from signal strength measurements, recovering the pose of rigid objects, deformable bodies, and articulated bodies from video sequences, and tracking a target in a completely uncalibrated network of sensors. For these tasks, this algorithm requires significantly fewer examples compared to fully-supervised regression algorithms or semi-supervised learning algorithms that do not take the dynamics of the output time series into account.
Description
Thesis (Ph. D.)--Massachusetts Institute of Technology, Dept. of Electrical Engineering and Computer Science, February 2006. Also issued as printed in pages. MIT Barker Engineering Library copy: printed in pages. Includes bibliographical references (leaves 113-119).
Date issued
2006Department
Massachusetts Institute of Technology. Department of Electrical Engineering and Computer SciencePublisher
Massachusetts Institute of Technology
Keywords
Electrical Engineering and Computer Science.