Case analysis studies of diffusion models on E-commerce transaction data
Author(s)
Lewis, Taariq; Long, Bryan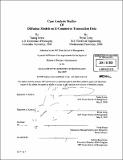
DownloadFull printable version (26.45Mb)
Other Contributors
Sloan School of Management.
Advisor
Vivek Farias.
Terms of use
Metadata
Show full item recordAbstract
As online merchants compete in the growing e-commerce markets for customers, attention to data generated from merchant and customer website interactions continues to drive ongoing online analytical innovation. However, successful online sales forecasting arising from historical transaction data still proves elusive for many online retailers. Although there are numerous software and statistical models used in online retail, not many practitioners claim success creating accurate online inventory management or marketing effectiveness forecast models. Thus, online retailers with both online and offline strategies express frustration that although they are able to predict sales in their offline properties, even with substantial online data, they are not as successful with their online-stores. This paper attempts to test two analytical approaches to determine whether reliable forecasting can be developed using already established statistical models. Firstly, we use the original Bass Model of Diffusion and modify it for analysis of online retail data. Then, we test the model's forecasting effectiveness to extrapolate expected sales in the following year. As a second method, we use statistical cluster analysis to categorize groups of products into distinct product performance groups. We then analyze those groups for distinct characteristics and then test whether we can forecast new product performance based on the identified group characteristics. (cont.) We partnered with a medium-sized online retail e-commerce firm with both online and offline retail channels to provide us with online transaction data. Using a modified Bass Diffusion Model, we were able to fit a sales forecast curve to a sample of products. We then used k-means cluster analysis to partition products into similar groups of sales transaction-behavior, over the period of 1 year. For each group, we tried to identify characteristics which we could use to forecast new product launch behavior. However, lack of accurate, characteristic mapping of products made it difficult to establish confidence in cluster forecasting for some groups with similar curves. With more accurate characteristic mapping of products, we're hopeful that cluster analysis can reasonably forecast new product performance in online retail catalogs.
Description
Thesis (M.B.A.)--Massachusetts Institute of Technology, Sloan School of Management, 2009. Includes bibliographical references (p. 50).
Date issued
2009Department
Sloan School of ManagementPublisher
Massachusetts Institute of Technology
Keywords
Sloan School of Management.