Learning Models of Sequential Decision-Making without Complete State Specification using Bayesian Nonparametric Inference and Active Querying
Author(s)
Unhelkar, Vaibhav V.; Shah, Julie A.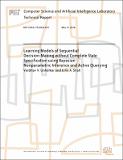
DownloadMIT-CSAIL-TR-2018-015.pdf (238.6Kb)
Other Contributors
Interactive Robotics Group
Advisor
Julie A Shah
Metadata
Show full item recordAbstract
Learning models of decision-making behavior during sequential tasks is useful across a variety of applications, including human-machine interaction. In this paper, we present an approach to learning such models within Markovian domains based on observing and querying a decision-making agent. In contrast to classical approaches to behavior learning, we do not assume complete knowledge of the state features that impact an agent's decisions. Using tools from Bayesian nonparametric inference and time series of agents decisions, we first provide an inference algorithm to identify the presence of any unmodeled state features that impact decision making, as well as likely candidate models. In order to identify the best model among these candidates, we next provide an active querying approach that resolves model ambiguity by querying the decision maker. Results from our evaluations demonstrate that, using the proposed algorithms, an observer can identify the presence of latent state features, recover their dynamics, and estimate their impact on decisions during sequential tasks.
Date issued
2018-05-17Series/Report no.
MIT-CSAIL-TR-2018-015
Keywords
Decision Making, Graphical Models, Human-AI Collaboration